Session 3 | AI & Emerging Technology
Wednesday, September 4, 2024 |
2:00 PM - 3:35 PM |
Speaker
Ms Liezel Viljoen
Member
AusIMM Southern Queensland Student Chapter
Session 3 | Keynote Introduction
2:00 PM - 2:05 PMBiography
Liezel is currently studying her Bachelor of Mining and Geotechnical Engineering at the University of Queensland located in St. Lucia, Brisbane.
Pat Boniwell
Managing Director - Australia
Byrnecut
The road ahead: Pat Boniwell on innovation
2:05 PM - 2:35 PMBiography
Pat Boniwell joined Byrnecut after graduating from WASM in 1995. He has since gained extensive experience in all aspects of underground mine development, production, construction and shaft sinking.
Pat’s experience covers a wide variety of underground operations across all major commodities and mining companies throughout Australia and New Zealand. Managing Director of Byrnecut since 2013, he was previously General Manager and Operations Manager.
In addition to holding a Bachelor of Engineering (Mining), he also holds an MBA from Melbourne Business School and a First Class Mine Managers Certificate of Competency.
Ms Liezel Viljoen
Member
AusIMM Southern Queensland Student Chapter
Session 3 | Keynote Q&A
2:35 PM - 2:50 PMBiography
Liezel is currently studying her Bachelor of Mining and Geotechnical Engineering at the University of Queensland located in St. Lucia, Brisbane.
Mr Luke Mohren
Student
Curtin University: Western Australian School of Mines
Applications of Artificial Intelligence for mineral processing in the mining industry
2:50 PM - 3:00 PMPresentation Overview
The integration of Artificial Intelligence (AI) in mineral processing automation and the mining industry holds transformative potential, enhancing various operational facets. One critical application of AI is in the size reduction of ores, an essential process for achieving uniform mineral distribution. By employing AI to monitor and adjust the size of rocks entering the mill, the process becomes more efficient and effective, leading to improved downstream processing and higher quality outputs.
In the realm of equipment maintenance, AI's role in predictive maintenance is invaluable. Traditional maintenance schedules often lead to unnecessary downtime or catastrophic equipment failures. AI mitigates these issues by continuously monitoring equipment health and performance. By analysing sensor data and identifying patterns indicative of wear and tear, AI can predict when maintenance is genuinely needed, ensuring that assets are taken offline only when necessary. This approach minimizes downtime, reduces maintenance costs, and extends the lifespan of critical machinery.
AI also excels in autonomous decision-making, which significantly reduces the need for human intervention. AI's ability to process and evaluate large datasets rapidly is a gamechanger as it can adjust equipment settings or reroute materials in real-time to optimize efficiency. This capability extends to analysing data from multiple sources to make informed decisions about ore extraction, resource allocation, and energy consumption. For instance, AI can dynamically adjust the operational parameters of crushers and mills to optimize throughput and energy usage, ensuring that the process remains both efficient and cost-effective.
Overall, the adoption of AI in mineral processing and mining stands to revolutionize the industry. AI-driven automation and decision-making enhance operational efficiency, reduce costs, and improve safety by minimizing human exposure to hazardous conditions. Furthermore, AI's advanced data analysis capabilities facilitate the discovery of new resources, ensuring the industry's sustainable growth. By leveraging AI, mining companies can achieve significant competitive advantages, leading to a more innovative and productive future for the sector.
In the realm of equipment maintenance, AI's role in predictive maintenance is invaluable. Traditional maintenance schedules often lead to unnecessary downtime or catastrophic equipment failures. AI mitigates these issues by continuously monitoring equipment health and performance. By analysing sensor data and identifying patterns indicative of wear and tear, AI can predict when maintenance is genuinely needed, ensuring that assets are taken offline only when necessary. This approach minimizes downtime, reduces maintenance costs, and extends the lifespan of critical machinery.
AI also excels in autonomous decision-making, which significantly reduces the need for human intervention. AI's ability to process and evaluate large datasets rapidly is a gamechanger as it can adjust equipment settings or reroute materials in real-time to optimize efficiency. This capability extends to analysing data from multiple sources to make informed decisions about ore extraction, resource allocation, and energy consumption. For instance, AI can dynamically adjust the operational parameters of crushers and mills to optimize throughput and energy usage, ensuring that the process remains both efficient and cost-effective.
Overall, the adoption of AI in mineral processing and mining stands to revolutionize the industry. AI-driven automation and decision-making enhance operational efficiency, reduce costs, and improve safety by minimizing human exposure to hazardous conditions. Furthermore, AI's advanced data analysis capabilities facilitate the discovery of new resources, ensuring the industry's sustainable growth. By leveraging AI, mining companies can achieve significant competitive advantages, leading to a more innovative and productive future for the sector.
Biography
Luke Mohren is a motivated and adaptable engineering student with a keen interest in the mining industry. Currently pursuing a Bachelor of Engineering in Chemical Engineering and a Bachelor of Science in Extractive Metallurgy at Curtin University, Luke has demonstrated academic excellence and leadership skills throughout his education. He has received numerous awards, including the Curtin Excellence Scholarship and the ADF Future Innovators Award. Luke's professional experience includes roles as a vacation student at Northern Star Resources, where he gained practical knowledge in safety procedures and chemical handling. He has also served as a martial arts instructor, labourer, and food and beverage attendant, honing his teamwork and problem-solving abilities. Luke is actively involved in the community at Curtin University as a member of the Curtin Engineering Club, WASM Wallabies Mining Games, and AusIMM Orediggers, allowing him to learn from his peers and form meaningful connections. His dedication to learning and innovation makes him a valuable asset in the mining sector.
Ms Sofiya Khan
RMIT Representative
AusIMM Melbourne Student Chapter
How harnessing caution against AI & technology can bolster positive public opinion & community outreach
3:00 PM - 3:10 PMPresentation Overview
The motivation behind this research stems from the growing distrust and criticism that the Australian mining sector receives across media outlets. Despite technological advancements aimed at enhancing efficiency and safety there is a persistent and notable disconnect in public perceptions and community engagement efforts. This research seeks to explore alternative approaches that can bridge this gap and improve the industry's public image to engineer multiple positive outcomes.
The research problem exposes the challenges that a technology-driven approach brings, in marketing and public relations’ content, in the face of building positive perceptions and community relationships. Findings suggest that traditional mining practices, like manual techniques and local employment, can potentially be effective in driving community engagement and advancing national pride.
The implications of this research are twofold. Firstly, it underscores the importance of diversifying approaches to community engagement beyond technological solutions. Secondly, it suggests that mining companies should prioritize the preservation and promotion of traditional practices in marketing and community outreach efforts to build a better sense of trust to improve public perceptions.
The research problem exposes the challenges that a technology-driven approach brings, in marketing and public relations’ content, in the face of building positive perceptions and community relationships. Findings suggest that traditional mining practices, like manual techniques and local employment, can potentially be effective in driving community engagement and advancing national pride.
The implications of this research are twofold. Firstly, it underscores the importance of diversifying approaches to community engagement beyond technological solutions. Secondly, it suggests that mining companies should prioritize the preservation and promotion of traditional practices in marketing and community outreach efforts to build a better sense of trust to improve public perceptions.
Biography
Sofiya Khan is highly accomplished across public relations, commerce, international relations, and the environmental sector. She is the recipient of the global Icon Agency FCF Scholarship, appeared on the Triple J, and currently sits on the Academic Board for RMIT. Sofiya has extensive leadership experience with being a Netball Victoria Board Observer, a Hub Manager for the Australian Crisis Simulation Summit (ACSS) and the Co-President for the Australian Business Student Association (ABSA) that oversees 12 member universities.
Ms Khan is committed to uplifting youth perspectives. She's an advocate for sustainable practices and enhances stakeholder engagement through her diverse experiences in academia. Sofiya is a part of multiple advisory groups such as but not limited too NYSO for the National Association Against Child Neglect & Abuse (NAPCAN) and Youth Affairs Council Victoria’s Biodiversity Youth Expert Advisory Group that involved working with the Department of Environment, Land, Water and Planning (DEECA). Sofiya recently finished public service as a Communications & Stakeholder Relations Intern for the North East Link which is the biggest ever investment in Melbourne’s north east. Now, Sofiya Khan is keen on sharing her interdisciplinary knowledge and creative thinking to accelerate decarbonisation efforts and advance sustainability, in the mining sector.
Mr Val Salamakha
Graduate Mining Engineer
Alkane Resources
Machine learning assisted lithology classification of drillhole data
3:10 PM - 3:20 PMPresentation Overview
In mineral exploration, accurate lithology classification of drillhole data is paramount for assessing geological features and enhancing the profitability of mining operations. Traditional methods rely heavily on a geologists’ visual inspection and subjective interpretation of core samples, which can be time-consuming and inconsistent. Machine learning algorithms applied in this classification context, have the capability to assist in these challenges by producing rapid first-pass interpretations even when the core samples are missing or damaged (Horrocks, Holden and Wedge, 2015).
The main objective of this study is to apply a variety of machine learning methods to conduct supervised classification of lithology types using multivariate geophysical data that is recorded during drilling operations. A total of 11 drillholes and four distinct lithology types were utilised in the application of each method. The data was submitted to training, cross-validation, and testing in three different scenarios using Decision Tree (DT), Random Forest (RF), Multi-layer Perceptron (MLP), Support Vector Classifier (SVC), XGBoost and LightGBM algorithms. A novel approach to crossvalidation was employed during the optimisation of each machine learning algorithm to preserve the sequential integrity of each drillhole. Balanced accuracy or macro-average recall was the primary evaluation metric for each algorithm.
The analysis revealed that while most algorithms can easily achieve over 90% balanced accuracy within the same drillhole, their performance declined by approximately 30% when applied to independent and previously unseen drillholes. A small increase in performance was observed in the same context when the models were trained with an expanded set of four drillholes. Among the tested algorithms, RF and SVC consistently outperformed the field on average across all testing scenarios. These results imply that access to a more extensive dataset, featuring consistent geological assessments with higher confidence intervals, may significantly improve each algorithms’ capability to classify lithology accurately and rapidly.
The main objective of this study is to apply a variety of machine learning methods to conduct supervised classification of lithology types using multivariate geophysical data that is recorded during drilling operations. A total of 11 drillholes and four distinct lithology types were utilised in the application of each method. The data was submitted to training, cross-validation, and testing in three different scenarios using Decision Tree (DT), Random Forest (RF), Multi-layer Perceptron (MLP), Support Vector Classifier (SVC), XGBoost and LightGBM algorithms. A novel approach to crossvalidation was employed during the optimisation of each machine learning algorithm to preserve the sequential integrity of each drillhole. Balanced accuracy or macro-average recall was the primary evaluation metric for each algorithm.
The analysis revealed that while most algorithms can easily achieve over 90% balanced accuracy within the same drillhole, their performance declined by approximately 30% when applied to independent and previously unseen drillholes. A small increase in performance was observed in the same context when the models were trained with an expanded set of four drillholes. Among the tested algorithms, RF and SVC consistently outperformed the field on average across all testing scenarios. These results imply that access to a more extensive dataset, featuring consistent geological assessments with higher confidence intervals, may significantly improve each algorithms’ capability to classify lithology accurately and rapidly.
Biography
Valentin Salamakha is a highly accomplished graduate of the University of New South Wales, holding a Bachelor of Mining Engineering (Honours) with First Class Honours and a Bachelor of Computer Science (Artificial Intelligence) with Distinction, both completed in 2023. Currently, Val is employed as a Graduate Mining Engineer with Alkane Resources, where he is contributing to the company's Tomingley Gold Mine operation.
During his academic career, Val was recognised for his outstanding achievements, notably receiving the prestigious Behre Dolbear Prize from the UNSW School of Minerals and Energy Resources Engineering. This award was granted for the best Honours Thesis related to Mining Engineering.
In addition to this, he has demonstrated strong leadership abilities as the Student Chapter President of the AusIMM Sydney Student Chapter in 2023. In this role, he organised events, fostered industry connections, and supported fellow students in their professional development.
With a solid foundation in both mining engineering and computer science, Val is well-equipped to tackle the challenges of the mining industry and drive innovation in this field.
Ms Liezel Viljoen
Member
AusIMM Southern Queensland Student Chapter
Session 3 | Abstract Q&A
3:20 PM - 3:30 PMBiography
Liezel is currently studying her Bachelor of Mining and Geotechnical Engineering at the University of Queensland located in St. Lucia, Brisbane.
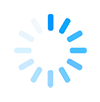